Cambridge EnerTech's
Battery Intelligence for Automotive Applications
(自動車向けバッテリーインテリジェンス)
材料から製造までの電池開発の最適化に向けた機械学習・人工知能の活用
2023年12月13日〜14日
電池市場の急速な成長と共に、その生涯性能を最適化する必要性も急速に拡大しています。自動車メーカーや電池パックメーカー、EVフリート管理者などにとって、電池の寿命を延ばすカギはデータにあります。機械学習やデータ分析の手法を活用すれば、電池データの潜在力を引き出し、電池の寿命を正確に測定・予測・改善することができます。人工知能が電池の技術空間に破壊的影響をもたらすため、電池の効率性や運用上の信頼性を高めるには、予見的インテリジェンスやデータ分析が重要な役割を果たします。自動車向けバッテリーインテリジェンスの部会では、業界のオピニオンリーダーや研究者が集結して、電池の寿命を大幅かつ継続的に改善するために、各企業がバッテリーインテリジェンスをどう活用すれば良いのかについて議論します。
12月13日(水)
Registration Open10:30 am
Organizer's Welcome Remarks2:00 pm
Victoria Mosolgo, Conference Producer, Cambridge EnerTech
BATTERY INTELLIGENCE IN AUTOMOTIVE SYSTEMS
自動車システムにおけるバッテリーインテリジェンス
Battery Health Monitoring: Integrating Data Analytics, Modelling Techniques, and Anomaly Detection for Enhanced Electric Vehicle Performance
Nikolaus Keuth, PhD, Senior Group Product Manager, IODP XI Data Analytics Solutions, AVL List GmbH
In today's and future sustainable mobility, electric powertrains play a pivotal role. Among all the components of electric vehicles, the battery holds the highest value. For manufacturers and mobility providers, the competition is determined by the total cost of ownership (TCO). To balance the reduction of TCO and battery costs while ensuring optimal performance, range, efficiency, and most importantly, lifetime, the continuous monitoring of the battery during operation is inevitable.
Overcoming Battery Hurdles through Software & ML/AI
Fabrizio Martini, Co-Founder & CEO, Electra Vehicles, Inc.
Many organizations, including Electra Vehicles, are using AI/ML to deliver greater business value as the ever-evolving technology plays an increasingly vital role in data communication and management. Join us to learn how Electra’s Adaptive Digital Twin technology works with the power of AI/ML to unlock the next big innovations that are moving the energy and EV industries forward at record pace.
Session Wrap-Up
Refreshment Break in the Exhibit Hall with Poster Viewing (Sponsorship Opportunity Available)3:25 pm
LIFETIME PREDICTIONS
電池寿命の予測
Machine Learning-Based Lifetime Prediction and Charging Optimization of Lithium-ion Batteries
Richard D. Braatz, PhD, Edwin R. Gilliland Professor, Chemical Engineering, Massachusetts Institute of Technology
This presentation will describe advances in machine learning-based techniques for addressing systems problems that arise for lithium-ion batteries. The specific systems problems include the prediction and classification of battery cycle lifetime (aka remaining useful life) and the determination of optimal charging protocols. The development of the techniques and their application are in collaboration with materials science, physics, and computer science researchers at Stanford University, Toyota Research Institute, and MIT.
Uncertainty-Aware and Explainable Machine Learning for Early Prediction of Battery Degradation Trajectory
Arghya Bhowmik, PhD, Assistant Professor, Energy Conversion & Storage, Danish Technical University
We demonstrate an early prediction model with reliable uncertainty estimates, which utilizes an arbitrary number of initial cycles to predict the whole battery degradation trajectory. Our model will enable accelerated battery development via uncertainty-guided truncation of cell cycle experiments once the predictions are reliable.
Special Issue on Physics-Informed Machine Learning Enabling Fault Feature Extraction and Robust Failure Prognosis
David A. Howey, PhD, Professor, Engineering Science, University of Oxford
The use of machine learning (ML) techniques has resulted in fairly high accuracy in diagnosing faults and predicting failures for a wide range of engineered systems. While these models can work well on often small quantities of fault/failure scenarios that a training dataset has captured, they may fail to perform on so-called out-of-distribution cases that are not well-represented in the training dataset but are encountered in real-world system operations. Advances in the understanding of how to incorporate physical knowledge into data-driven ML models have the promise of making these models generalize better to unseen, out-of-distribution cases while at the same time explaining underlying physics.
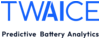
Stephan Rohr, PhD, Co-CEO, TWAICE Battery Analytics Platform
The demand for actionable insights on battery pack health, safety, and aging in the mobility sector is growing exponentially. TWAICE Battery Health Package uses in-life data to assess a battery's state, eliminating costly check-up procedures. I will highlight the challenges we faced to provide a 2% performance guarantee on our SoH estimates, while covering a range of different battery types, sensor availabilities, data resolutions, and qualities of in-life mobility data.
Close of Day5:50 pm
12月14日(木)
Registration and Morning Coffee8:30 am
Organizer's Remarks9:00 am
DIAGNOSTICS & MODELING
診断・モデリング
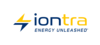
Daniel Higgs, PhD, Director of Revenue, Business Development, Iontra Inc
This presentation will discuss why most advanced charging technologies fall short of fully maximizing the performance of today's batteries. We will present a case for the need to understand how current flows through a battery system and why this is critical for a cost-effective and scalable advanced charging solution that achieves double the charge speeds (without negatively affecting cycle life).
Fast and Reliable SOH Estimation with a Hybrid Diagnostic Algorithm
Yeong Yoo, Research Officer, Energy Mining & Environment Research Center, National Research Council Canada
A fast, accurate, and reliable SOH diagnostic algorithm based on a combination of time-domain diagnostics, parameterization, and machine learning (ML)-based regression and optimization will be presented. This unique algorithm can be utilized for a variety of applications such as mobile, stationary, marine, aviation, and EVSE, etc.
Advances in Multiscale Modelling of Electrochemical Systems
Venkat R. Subramanian, PhD, Ernest Dashiell Cockrell Chair Engineering, Professor, Mechanical & Materials Science Engineering, University of Texas Austin
Model-based Battery Management System (BMS) can be used to improve the performance of current and next-generation lithium batteries. Recent results for life improvement, reduction in charging time for cells, packs, and modules, and next-generation batteries will be presented. The importance of physics-based electrochemical models, suitable algorithms, and experimental validation will be discussed.
Session Wrap-Up
Mona Faraji-Niri, PhD, Assistant Professor, Energy Systems, Energy Innovation Centre, University of Warwick
Yeong Yoo, Research Officer, Energy Mining & Environment Research Center, National Research Council Canada
Coffee Break in the Exhibit Hall with Poster Viewing10:45 am
BATTERY INTELLIGENCE FOR MANUFACTURING AND BEYOND
バッテリーインテリジェンス:製造工程とその先に向けて
Battery Intelligence: Why You Can’t Afford to Wait
Eli Leland, PhD, CTO and Co-Founder, Voltaiq, Inc.
It’s go time for the battery sector. Companies across the value chain are racing to secure materials supply, ship new products, ramp new gigafactories, and optimize post-sale value for customers. Missteps at this stage can mean delayed revenue, costly reengineering, or safety incidents that can risk lives and trigger huge recalls. To succeed, companies must learn faster than the competition. Many organizations now understand that enterprise battery intelligence offers a path to world-class battery excellence, but consider it a nice-to-have until more urgent initiatives are addressed. This talk will illuminate how companies can’t afford to wait, as battery intelligence delivers immediate ROI and is in fact the key to accelerating in the midst of developing supply chains, evolving chemistries, and ever more demanding applications.
Explainable Machine Learning for Lithium-ion Battery Manufacturing
Mona Faraji-Niri, PhD, Assistant Professor, Energy Systems, Energy Innovation Centre, University of Warwick
Using AI and ML for lithium-ion battery modeling while answering questions regarding the predictability and accuracy of the models at various at stages of manufacturing, leave the manufacturer with a black box model hard to explain. The XAI and XML have a significant roll in shedding some light on the model by explaining how the decision is made and how the values are predicted.
Cut Battery Development Time and Cost through Virtual Testing and Digital Labs
Gerald Sammer, PhD, Principal Business Development Manager, Integrated & Open Development Platform, AVL List GmbH
How can the time-to-market of new batteries and electrical vehicles shortened? Testing hundreds or thousands of batteries and cells simultaneously in a multi-vendor lab is a huge challenge and time consuming. Efficient processes and an open toolchain for automated scheduling, monitoring, energy management, and data analytics are the key to success as part of that virtual testing can cut development time and cost, e.g. with model based cell aging prediction or AI based battery fleet data modelling.
Networking Lunch (Sponsorship Opportunity Available)1:05 pm
Dessert Break in the Exhibit Hall with Poster Viewing - Last Chance for Viewing (Sponsorship Opportunity Available)2:00 pm
MODELING
モデリング
Integrating Physics-Based Modelling with Machine Learning for Lithium-ion Batteries
Huazhen Fang, PhD, Associate Professor, Mechanical Engineering, University of Kansas
Despite their merits, lithium-ion batteries still face significant performance and safety bottlenecks. Physics-informed machine learning has proven as a useful way to make batteries work better, and live up to their potential. In this talk, we will share our explorations on this topic, especially for modelling and condition monitoring for lithium-ion battery systems. We will further discuss prospective opportunities and challenges.
Battery Health Prognostics with Transfer Learning
Yunhong Che, PhD, Assistant Professor, Aalborg University
Health prognostics are essential for smarter battery management. It is difficult to guarantee performance under disparate distributions of lifetime and degradation patterns however, due to a variety of battery types and application scenarios. The effectiveness of various transfer learning strategies for performance improvement in battery health prognostics will be discussed in light of the accessibility of operating data and capacity labels. The fundamental concept and the applicability of various transfer learning strategies will be shown. Specifically, the sparsely labeled data fine-tuning, unsupervised domain adaptation, and no source labeled data condition-driven self-supervised strategy will be discussed in this presentation.
Sponsored Presentation (Opportunity Available)3:15 pm
Networking Refreshment Break3:50 pm
BATTERY MANAGEMENT
電池管理
Advanced Battery Management System for EV Application
Saeid Habibi, PhD, Professor Mechanical Engineering, Center for Mechatronics & Hybrid Technologies, McMaster University
This study presents a comprehensive review of the latest developments and technologies in battery characterization and their application in Battery Management Systems (BMS) for Electric Vehicles (EV).
Pulse Injection-Aided Machine Learning for Battery Pack State Estimation
Matthias Preindl, PhD, Assistant Professor, Electrical Engineering, Columbia University
Lithium-ion (LIB) battery degradation is often characterized at three distinct levels: mechanisms, modes, and metrics. ML can provide a unique multi-level perspective on characterizing LIB degradation and it can improve accuracy especially when combined with perturbation techniques. This pulse injection aided machine learning (PIAML) technique can be used for battery diagnostic and prognostics with high fidelity. LIB management systems can leverage this estimation framework to extend lifetime and reduce costs. Battery degradation: impedance and incremental capacitance. Estimation of mechanisms, modes, and metrics including SoC, SoH, SoP.
Close of Conference4:55 pm
* 不測の事態により、事前の予告なしにプログラムが変更される場合があります。
アジェンダ・講演者・スポンサー更新

