
Cambridge Healthtech Instituteの第7回年次
AI/Machine Learning for Early Drug Discovery – Part 2
早期創薬向けAI/ML – パート2
AI/ML for Exploring and Screening Complex Target Biology and Chemical Space
複雑なターゲット生物学と化学空間の探究とスクリーニング向けAI/ML
2025年4月16日 - 17日PDT(米国太平洋標準時)
「早期創薬向けAI/ML」は2部構成の会議で、化学、標的発見、薬理学、バイオインフォマティクスの多様な専門家が集まり、医薬品開発における計算ツール、モデル、アルゴリズム、データアナリティクスの利用拡大について話し合います。会議では、低分子・ペプチドの医薬品開発に関連するケーススタディを用いて、AI/MLドリブンの意思決定における長所と短所に焦点を当てます。会議の第1部では、医薬品設計、ヒットの同定、PK/PD・薬物様の特性の予測、リードの最適化に、AI/MLがどのように役立つかに焦点を当てます。第2部では、ターゲットの同定、細胞経路のデコンボリューションのための新興の計算ツールやモデルに焦点を当て、様々な治療領域の化学的空間の検証によってニッチなアプリケーションを促進することができます。
4月16日(水)
12:00 pmRegistration Open
AI/ML FOR PEPTIDE & ANTIBODY OPTIMIZATION
ペプチド・抗体の最適化向けAI/ML
Peptide Hit Discovery and Optimization Using Machine Learning and Small Peptide Arrays
Ewa Lis, PhD, Founder & CEO, Koliber Biosciences
Peptide discovery methods like phage and mRNA display are standard tools but face challenges such as high false positive rate or costly licensing, limiting novel therapeutic discovery. We present a new platform combining Koliber’s machine learning technology with RobustDx's peptide array technology, demonstrating that large libraries are unnecessary and that hits can be efficiently optimized to nanomolar binding affinity. Additionally, we showcase visualization techniques for binding mode detection and offer insights into the future of machine learning-driven peptide optimization.
AlphaBind, a Domain-Specific Model to Predict and Optimize Antibody-Antigen Binding Affinity
Ryan Emerson, PhD, Vice President, Data Science, A Alpha Bio Inc.
We present AlphaBind, a domain-specific model achieving state-of-the-art performance in optimizing antibody affinity using protein language model embeddings and extensive pre-training. We demonstrate affinity optimization for four antibodies with just one round of training data generation per antibody, and we demonstrate the use of a fine-tuned AlphaBind model to guide downstream engineering for biodevelopability and germline reversion for one antibody. AlphaBind weights and code are publicly available.
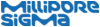
Suhasini Murali Iyengar, Application and Discovery Scientist, AI and Cheminformatics, MilliporeSigma
In collaboration with the Drugs for Neglected Diseases initiative (DNDi), we used predictive ML ADMET models via the AIDDISON™ software to profile compounds from a lead series in an open-science anti-Chagas drug discovery program. The goal was to identify scaffold hopping opportunities with improved druglike properties to advance this crucial program. Using top-scoring compounds from a multi-parameter ADMET profile, we conducted a pharmacophore search of ultra-large, virtual chemical space and ran generative chemistry calculations to discover promising new leads.
3:10 pmBreakout Discussions (In-Person Only)
Breakout Discussions are informal, moderated discussions, allowing participants to exchange ideas and experiences and develop future collaborations around a focused topic. Each breakout will be led by a facilitator/s who keeps the discussion on track and the group engaged. Please visit the Breakout Discussions page on the conference website for a complete listing of topics and descriptions. Breakout Discussions are offered in-person only.
3:55 pmRefreshment Break in the Exhibit Hall with Poster Viewing
Unlocking Challenging Cardio-Metabolic Targets for Antibody Drug Discovery with Protein Engineering and Design
Alexander Taguchi, PhD, Director, Machine Learning, Antibody Discovery, iBio, Inc.
Cardio-metabolics as a therapeutic area has surged in interest, but the tools to tackle this space for antibody drug discovery remain underdeveloped. Here we describe a machine learning protein design platform for efficient antibody discovery against the TGFβ superfamily. This approach produces epitope-specific antibodies even when the target of interest is unavailable for screening. This is made possible by leveraging machine learning to design (1) soluble protein representations of the target for epitope-selective antibody discovery and (2) antibody libraries to optimize the paratope space for high-value cardio-metabolic targets.
5:45 pmClose of Day
5:45 pmDinner Short Course Registration
6:15 pmDinner Short Course*
SC7: AI Applications in Drug Development: Strategies for Innovation and Integration
*Premium Pricing or separate registration required. See Short Courses page for details.
4月17日(木)
7:15 amRegistration Open
7:45 amBreakfast Panel Discussion: Diversity in Chemistry (People, Not Molecules) (Sponsorship Opportunity Available)
Grab a plate and seat (breakfast provided by Drug Discovery Chemistry) to join a dynamic discussion about growing the enterprise of chemistry. This session originated with a focus on "Women in Chemistry," but every year the discussion, guided by audience interest and participation, evolves. This panel discussion is being offered in-person only.
PANEL MODERATOR: Michelle Arkin, PhD, Chair and Distinguished Professor, Pharmaceutical Chemistry & Director, Small Molecule Discovery Center, University of California, San Francisco
PANELISTS: Tom Garner, Genentech; Katerina Leftheris, formerly Vilya Therapeutics; Justyna Sikorska, Merck
- Do family leave policies at companies impact men and women equally?
- Where does the 'drop-off' of women in the chemistry career progression pipeline occur and why?
- What tools does your institution use, or do you wish for, to help increase diversity in gender or under-represented races.
PLENARY KEYNOTE SESSION
プレナリーセッション(基調講演)
Simplifying Synthesis with Radicals
Phil Baran, PhD, Chair & Professor, Department of Chemistry, Scripps Research Institute
Our latest findings on how the use of radical cross-coupling can dramatically simplify the practice of medicinal chemistry will be presented through the invention of reactions that have wide-substrate scope, use ubiquitous starting materials, and are experimentally trivial to conduct.
9:25 amCoffee Break in the Exhibit Hall with Poster Viewing and Best of Show Awards Announced
AI-BASED SCREENING FOR TARGETS & LEADS
ターゲット・リード向けAIベースのスクリーニング
Ultrafast Screening and Optimization in Allosteric Pockets with 3D/AI-CPU/GPU Pipeline: Flavivirus Proteases and More
Ruben Abagyan, PhD, Professor, Skaggs School of Pharmacy and Pharmaceutical Sciences, University of California, San Diego
Finding the first potent and selective inhibitors against a transient, allosteric, or protein-protein interaction pocket is a challenge requiring multiple levels of data, tools, profile definitions, and ultra large screens combined with in silico compound optimization. We present a cloud-based CPU/GPU pipeline designed for that purpose and its application for identifying drug candidates among multibillion compounds. Examples with anti-cancer targets and inhibitors of anti-flaviviral proteases are presented.
Novel AI-Based Methods for Ultra-Large and Ultra-Fast Virtual Screening in Drug Discovery
Leif Eriksson, PhD, Professor, Chemistry & Molecular Biology, University of Gothenburg
The druglike chemical space of available molecular databases contains ~10¹ molecules, and grows faster than traditional screening approaches can handle. We present benchmarked methods that circumvent conformational sampling, enabling ultra-large and ultra-fast screening, including a novel AI-based scoring function, generative AI, and scaffold optimization. We also report on a data-driven molecular descriptor model using Neural Machine Translation, for effectively predicting protonation states, performing similarity searches, and generating molecular derivatives.
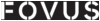
Fengbo Ren, CEO, Computer Science & Engineering, Fovus Corp
Fovus is an AI-powered, serverless high-performance computing (HPC) platform delivering intelligent, scalable, and cost-efficient supercomputing power at the computational scientists' fingertips. Fovus uses AI to optimize HPC strategies and orchestrates cloud logistics, making cloud HPC a no-brainer and ensuring sustained time-cost optimality for computational drug discovery amid quickly evolving cloud infrastructure. By accelerating time-to-insights and optimizing cloud costs, Fovus helps Biotech clients accelerate Design-Make-Test-Analyze (DMTA) cycles and discover more with less. Join this talk to learn how Fovus can supercharge your computational drug discovery with case studies and GROMACS/AlphaFold 3 benchmarking results.
AI-Driven Virtual Screening and Polypharmacology Analysis
Sita Sirisha Madugula, PhD, Postdoctoral Research Associate, Center for Nanophase Materials Sciences, Oak Ridge National Laboratory
Our research demonstrates the potential of AI and machine learning in drug repurposing, specifically for tuberculosis (TB). Through unsupervised learning and polypharmacology approaches, we identified FDA-approved drugs with potential for repurposing by analyzing molecular descriptors and multi-target interactions. These methods offer efficient pathways to explore chemical and biological spaces, providing new insights into drug efficacy and paving the way for therapeutic solutions in infectious and non-infectious diseases.
12:35 pmTransition to Lunch
12:40 pmLuncheon Presentation (Sponsorship Opportunity Available) or Enjoy Lunch on Your Own
1:10 pmDessert Break in the Exhibit Hall with Poster Awards Announced (Sponsorship Opportunity Available)
CHALLENGES INTEGRATING DIVERSE DATA
多様なデータ統合の課題
2:50 pmChairperson’s Remarks
Harmonizing Diverse Data Types and Sources for Drug Discovery and Machine Learning
Peter Canning, PhD, Principal Scientist, Protein & Structural Sciences, CHARM Therapeutics
Evidence has shown that the functional performance of many ML models improves with target-specific training data. We have established a platform to collect and organize various internal and external data sources to inform drug discovery projects and train ML models for improved output confidence. DragonFold is CHARM therapeutics’ state-of-the-art co-folding platform for prediction of ligand-bound protein structures.
AI Methods to Integrate Multi-Modal Omics, Spatial, and Single-Cell Profiling to Identify Mechanisms and Potential Therapeutic Opportunities
Arvind Rao, PhD, Associate Professor, Department of Computational Medicine and Bioinformatics, University of Michigan
Spatial profiling technologies coupled with scRNAseq enable a multi-factorial, multi-modal characterization of the tissue microenvironment. Objective scoring methods inspired by recent advances in statistics and ML can aid the interpretation of these datasets, as well as their integration with companion data like bulk and single-cell genomics. I will discuss analysis paradigms from ML that can be used to integrate and prioritize gene regulatory programs (and therapeutic candidates) underlying oncogenesis.
3:55 pmNetworking Refreshment Break
MACHINE-LEARNING & DNA-ENCODED LIBRARY TECHNOLOGY
ML・DELテクノロジー
Machine Learning for 3D-Aware Molecular Representations in DEL
Angelina Heyler, Data Scientist, Encoded Libraries, GSK
DNA-encoded libraries (DELs) enable screening billions of ligands against protein targets of interest. To select hits for off-DNA evaluation, quantitative structure-activity relationship (QSAR) modeling is frequently used to find structural features that contribute to enrichment. However, current QSAR typically relies on 2D molecular representations. We leverage machine learning to learn 3D molecular representations for application in hit selection.
Ligandability of WDR-Containing Proteins Using DEL Then ML
Peter J. Brown, PhD, Chemical Probes, University of North Carolina at Chapel Hill
Target class-focused drug discovery has a strong track record in pharmaceutical research, yet public domain data indicate that many protein families remain unliganded. Here we present a systematic approach to scale up the discovery and characterization of small molecule ligands for the WD40 repeat (WDR) protein family. A pilot hit-finding campaign using DNA-encoded chemical library selection followed by machine learning (DEL-ML) yielded first-in-class, drug-like ligands for 7 of the 16 WDR domains screened. This study establishes a template for evaluation of protein family ligandability and provides extensive WDR resources to discover ligands for this underexplored target class.
DELs in Medicinal Chemist's Toolbox: Applications beyond Hit Discovery
Kirill Novikov, PhD, Principal Scientist, High Throughput Chemistry, insitro
DNA-encoded libraries (DELs) are traditionally used to find potential hit compounds for specific targets. At insitro, we are enhancing this technology to aid in later stages of drug discovery. We employ targeted second-generation DELs for efficient exploration of chemical space surrounding hit structures. By employing affinity-based electrophoretic separations, such as nDexer and capillary electrophoresis, we can rank DEL members, facilitating early structure-activity relationship (SAR) hypothesis formation and machine-learning model training to refine predictive accuracy in this chemical environment. As a practical example, we will showcase the design and construction of three second-generation DELs: one based on a DEL hit, another on a literature compound, and the third on virtual docking.
5:40 pmClose of Conference
*不測の事態により、事前の予告なしにプログラムが変更される場合があります。
アジェンダ・講演者・スポンサー更新
2025年 4月 14日
2025年 4月 15 - 16日
2025年 4月 16 - 17日