Cambridge EnerTech’s
Battery Engineering
バッテリーエンジニアリング
Optimizing Cell and Pack Design through Engineering and AI
March 19-20, 2025
As battery technology progresses, there's a growing need for meticulously engineered battery systems that fully leverage active cell materials. It's essential to develop packs that ensure consistent cell performance and seamlessly integrate them into products, prioritizing safety, reliability, and durability. The design of cells, including the choice of non-active components, plays a significant role in battery performance and reliability. Designing and integrating battery packs present challenges in thermal, mechanical, and electrical engineering, regardless of cell chemistry. Achieving optimal cell and pack design tailored to the application's duty cycle requires a careful balance of energy and power capacity, manufacturability, abuse tolerance, thermal characteristics, and cost considerations. Battery Engineering will cover these topics as well as how artificial intelligence can help enhance battery performance.
3月19日(水)
Registration Open6:50 am
Networking Luncheon1:00 pm
Dessert Break in the Exhibit Hall with Poster Viewing2:15 pm
MATERIALS DESIGN
Air Power: Lithium-ion Batteries
Lane Crofton, R&D Research Engineer, SAFT
From F35 to F1: an overview of Saft Power Capability. Saft has established a market-leading position in providing batteries with very-high power capability. Recent efforts have led to an improvement in various dimensions
of Saft’s very-high power–capable products, making them even more well-suited for the applications in which they are used. A summary of the work undertaken and the performance advantages that have resulted is presented.
Computation Design for Materials
Chen Ling, PhD, Principal Scientist, Toyota Research Institute of North America
Lethargic oxygen evolution reaction (OER) hinders proton exchange membrane water electrolyzer adoption for green hydrogen production. While iridium- and ruthenium-based catalysts are prevalent, this study proposes a
high-throughput screening approach, predicting 61 potential acid OER candidates from 6912 pyrochlore compounds, including promising p-block metal dopants. These findings highlight pyrochlore compounds as versatile
materials for various applications.
Refreshment Break in the Exhibit Hall with Poster Viewing4:20 pm
BATTERY SYSTEMS AND TESTING
Lithium Battery Packs and Battery Management Systems for Racing Applications
Derek Barger, Chief of Electronics, Engineering, Doroni Aerospace
We will examine custom battery packs engineered for racing vehicles and explore how these innovations are being adapted for use in the aerospace industry, particularly in electric vehicle vertical takeoff and landing (eVTOL) aircraft. This discussion will cover their implications for safety, battery pack design, and the circular battery pack recycling system.
Fast Track to Superior Automotive Batteries: Virtual System Validation and AI-Driven Testing
Gerald Sammer, PhD, Principal Business Development Manager, AVL List GmbH
The proposal highlights the use of advanced AI and virtual testing methods to enhance battery performance and quality for automotive applications in much shorter time. By integrating state-of-the-art simulation tools
and virtualization solutions, this approach allows for rapid, cost-effective development and optimization of battery systems. Utilizing predictive modeling and iterative design optimization, engineers can explore
diverse scenarios, accelerating the development process and significantly improving quality and cycle-life of Li-ion batteries.
Big Data for the Diagnosis and Prognosis of Deployed Battery Systems
Matthieu Dubarry, PhD, Assistant Researcher, Battery Testing & Evaluation & Modeling, University of Hawaii
The diagnosis and prognosis of deployed batteries is complex because the cells might never experience controlled conditions during operation as both the charge and discharge duty cycles could be sporadic. To circumvent
this issue new methodologies must be implemented and thoroughly validated. This work presents a new methodology for diagnosis that used real observed solar irradiance, modeled clear sky irradiance, a load usage model,
and synthetically-generated battery data from a battery digital twin to diagnose the degradation of commercial Li-ion batteries connected to photovoltaic systems.
Close of Day6:30 pm
3月20日(火)
Registration and Morning Coffee8:00 am
TEMPERATURE CONTROL
Controlling the Temperature Build-Up in Electrode-Sealed Coin-Shaped Batteries via Designing Central Heat Dissipator
Stanislav I. Stoliarov, PhD, Professor, Fire Protection Engineering, University of Maryland
This talk explores innovative strategies for managing temperature build-up in electrode-sealed coin-shaped batteries. By designing an efficient central heat dissipator, we enhance thermal regulation, improving battery
performance and lifespan. Attendees will learn about the impact of thermal management on energy storage systems and the potential for increased safety and efficiency.
Battery Booth Crawl with Bagels in the Exhibit Hall with Last Chance for Poster Viewing9:00 am
AI BATTERY MANAGEMENT SYSTEMS

Johan Sundqvist, VP Sales, Sales, COMSOL Inc
Designing high-performance, durable, and safe batteries requires a detailed understanding of battery technology and the underlying physical processes. The physics-based modeling approach in COMSOL helps designers to create accurate simulations of batteries, thus reducing the need for time-consuming and expensive experiments. The COMSOL software provides modeling solutions of batteries ranging from simulating micro-structures within the battery’s porous electrode to the battery pack scale. In this presentation, we will explore various aspects of electrochemical modeling for battery cells, upscaling to system-level modeling and incorporating thermal management solutions for battery packs.
Attendee Transition to Booth Crawl in Exhibit Hall10:00 am
PREDICTING BATTERY DEGREDATION
A Method for Estimating the Useful Lifetime of Lithium-ion Battery
Cher-Ming Tan, PhD, Director, Center for Reliability Sciences & Technologies, Chang Gung University
Current method of useful life is based on the SoH monitoring of LiB and perform various methods to estimate from the trend of SoH degradation, and usually these LiB cells are already connected. This talk presents a
method where the estimation is done on the first charge-discharge cycle of LiB cell, allowing for good selection of cells for pack building. This method is verified experimentally using commerical LiB cells of different
rating from different suppliers. It can also help to spot used cells that may be sold as fresh cell. It can also apply to connected cells during their operations.
Predicting Rapid Degradation Onset in Lithium-ion Batteries during Real-Time Operation Using Machine Learning
Vikas Tomar, PhD, Faculty Lead, Innovation and Commercialization, School of Aeronautics and Astronautics, Purdue
This talk presents low data machine learning models for capacity degradation trajectory and EOL of commercial lithium-ion batteries (LIBs) using the data from the initial historical cycles, employing a recently developed machine learning approach based on a capacity degradation network (CD-Net). Additionally, the machine learning models are employed to predict the knee point in the degradation curve showing applicability to accident scenario. Finally, data is shown in real world operations.
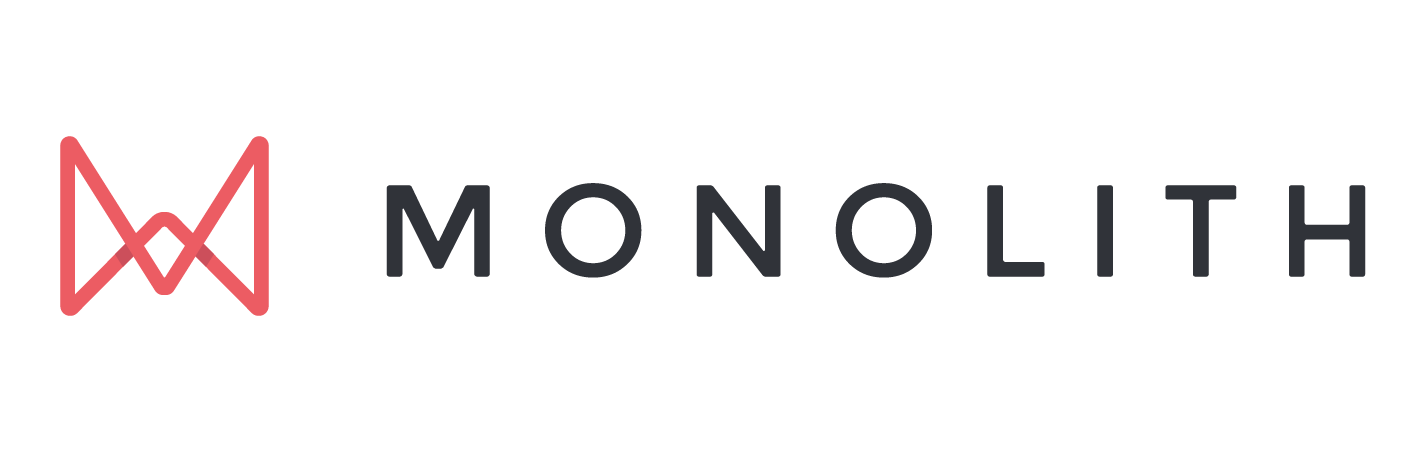
Can We Trust AI for Battery Design and Testing?
Richard Ahlfeld, CEO, Monolith AI
AI is transforming industries, offering powerful new tools but also raising debates around regulation and trust. In engineering, AI can streamline processes, enhance innovation, and reduce costs—but is it always appropriate?
In this talk, Richard Ahlfeld explores how AI and machine learning can drive faster time-to-market, improved design, and cost savings, with a focus on battery technology. Through practical examples, he’ll demonstrate AI’s impact on optimising battery performance, energy storage, and predictive maintenance, and discuss future opportunities and challenges for AI in engineering.
Enjoy Lunch on Your Own12:15 pm
CONTROLS & MODELLING
Model-Based Control and Machine Learning for Lithium-ion and Lithium-Sulfur Batteries
Hosam K. Fathy, PhD, Mechanical Engineering, University of Maryland College Park
This talk will provide a high-level exploration of some of the key challenges and opportunities in the model-based control of both lithium-ion and lithium-sulfur batteries, including both solid and liquid electrolyte
Li-S batteries. Much of the talk will focus on emerging opportunities for test trajectory optimization and machine learning for both battery types, with a focus on Li-S batteries.
Estimating Parameters of Physics-Based Model of Lithium-Metal Battery Cells Using EIS
Gregory L. Plett, PhD, Professor, Electrical & Computer Engineering, University of Colorado, Colorado Springs
Lithium-metal batteries hold promise for heavy-duty transportation due to their high energy density, but before they can be adopted, battery-management systems (BMS) must be developed to monitor and control their operation.
This talk introduces a method to parameterize a physics-based model of these cells for BMS application that uses simple tests performed on electrochemical-impedance-spectroscopy (EIS) equipment.
A Comparative Review of Simplified Battery Models Used for Advanced Controls
Scott Trimboli, PhD, Professor, Electrical & Computer Engineering, University of Colorado, Colorado Springs
State-of-the-art BMS rely on accurate battery models and specialized algorithms to obtain useful estimates of the battery state in order to ensure proper performance and safe operation. Most practical models are simplifications,
and thus must trade off high accuracy for computational efficiency. This talk examines the implications of using various simplified models in the performance of key BMS tasks.
Understanding, Modeling, Validating, and Predicting Lithium-ion Battery Degradation
Gregory J. Offer, PhD, Professor in Electrochemical Engineering, Imperial College London
This presentation covers understanding lithium-ion battery degradation, how to model it, and how close those models are getting to usefully predict lifetime. We will describe our efforts to model lithium plating, SEI
layer growth, positive electrode (cathode) decomposition, unequal degradation in silicon carbon composite electrodes, particle cracking, electrolyte consumption and cell dry-out, and how multiple degradation mechanisms
are coupled with each other and contribute towards accelerated degradation (the knee point/cliff-edge/etc).
Transition to Closing Plenary Panel3:15 pm
Close of Conference4:30 pm
* 不測の事態により、事前の予告なしにプログラムが変更される場合があります。
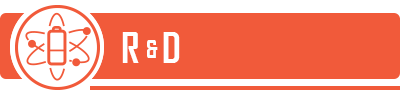
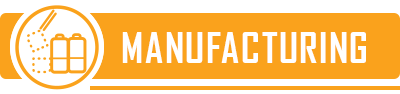
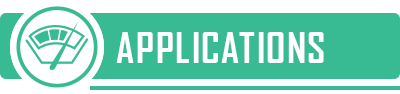
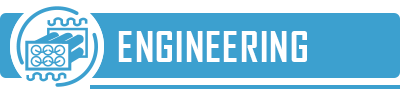