Cambridge Healthtech Instituteの第3回年次
ML and Digital Integration in Biotherapeutic Analytics
バイオ医薬品分析におけるMLとデジタル統合
Best Practices for Implementing and Optimizing Big Data Tools in the Analytical Function
分析機能におけるビッグデータツールの実装と最適化のベストプラクティス
2025年5月12日 - 13日 EDT(米国東部標準時・夏時間)
Sunday, May 11
1:00 pmMain Conference Registration
2:00 pmRecommended Pre-Conference Short Course
SC1: In silico and Machine Learning Tools for Antibody Design and Developability Predictions
*Separate registration required. See short course page for details.
Monday, May 12
7:00 amRegistration and Morning Coffee
TOOLS AND MODELS FOR ANALYTICAL SCIENTISTS
Enhancing Laboratory Analysis with Generative AI-Based Chatbot
Michail Vlysidis, PhD, Senior Engineer, AbbVie
In today's rapidly evolving technological landscape, the integration of advanced technologies such as large language models (LLMs) and generative AI has the potential to revolutionize laboratory analysis. We present a novel approach to enhancing laboratory information management systems (LIMS) through the integration of a knowledge-driven chatbot powered by LLMs. By leveraging internal databases, the chatbot is designed to provide specialized and tailored assistance to scientists, streamlining their workflow and improving overall efficiency. The chatbot utilizes the power of LLMs to understand user queries, retrieve relevant information from the database, and generate informative responses in a conversational manner.
Democratizing Data Analysis through Automation
Sara Byers, PhD, Principal Scientist, Quantitative Sciences & Digital Transformation, Bristol-Myers Squibb
Substantial data analysis is routinely performed to support critical decisions and filings. The process from analysis to reporting results requires time-consuming, repetitive manual effort. We will share our insights on scripted add-ins developed that take this process from hours to seconds. These tools can be distributed to empower scientists, increase development speeds, reduce human error, and streamline workflows, allowing us to focus on more strategic and innovative activities.
An Introduction to Machine Learning Lifecycle Ontology and Its Applications
Milos Drobnjakovic, Research Associate, Systems Integration, NIST
Machine Learning (ML) has shown promise in drug discovery and manufacturing, but its effective utilization faces hurdles across ML lifecycle including traceability, meeting regulatory requirements, siloing of related tools and their interoperability, model understanding, cross-organization collaboration, and dataset and model reuse. To address these hurdles, this presentation introduces the Machine Learning Lifecycle Ontology (MLLO), a standardized framework to capture ML metadata throughout the lifecycle. A MLLO-based prototype tool, ML Lifecycle Explorer, will be demonstrated. The talk concludes with a future work which will explore an additional MLLO potential to enhance model development and reuse by connecting it to domain knowledge.
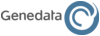
Jana Hersch, Head of Corporate Scientific Engagement, Genedata
Integrating machine learning (ML) in biotherapeutic analytics is a transformative journey poised to revolutionize drug development. It requires high-quality data, technological advancements coupled with scalability, and interdisciplinary collaboration. This talk will showcase four real-world examples of biopharma companies tackling strategic challenges in ML integration: bridging digitalization gaps in chromatography, automating HT mass spectrometry workflows, validating automated NGS analysis, and using ML-derived developability data in discovery workflows.
10:30 amNetworking Coffee Break
Analytical Toolkit for Structural Characterization with Mass Spec
Simon Letarte, PhD, Director, Extended Structural Characterization, Gilead Sciences Inc.
Protein characterization with mass spectrometry involves a series of tools. Most labs have Orbitraps and QTOFs. Methods include reduced and non-reduced peptide maps, intact and subunit mass, as well as native MS methods such as native SEC and native CEX. Multidimensional chromatography (2D-LC) are useful either for complex separations or using salt-based eluents on a mass spectrometer. It is desirable to have a cross-platform data processing suite that can open data files from all instrument manufacturers as well as specialized in-house software tools. Templated reports provide structure to your data for integration with systems such as ELN and ML/AI applications.
Predicting Subvisible Particle Formation of Monoclonal Antibodies Using Quartz Crystal Microbalance with Dissipation
Yibo Wang, PhD, Postdoctoral Fellow, Machine Learning, AstraZeneca
The occurrence of subvisible particles (SVPs) in monoclonal antibody (mAb) development presents challenges in assessing product stability. This study utilizes quartz crystal microbalance with dissipation (QCM-D) in silico and experimental physicochemical properties to investigate SVP formation risks associated with various containers and stress types. MAb adsorption kinetics were found to strongly correlate with SVP propensity in the stirring study, and in silico predictors significantly improved all model performance.
12:00 pmSession Break
12:10 pmLuncheon Presentation (Sponsorship Opportunity Available) or Enjoy Lunch on Your Own
1:10 pmSession Break
KEYNOTE PRESENTATION: AI in Biopharmaceutical Development: What Could Go Wrong?
Christopher P. Calderon, PhD, Associate Research Professor, Chemical and Biological Engineering, University of Colorado
This presentation presents an overview of recent practical challenges associated with designing and using data-driven algorithms in pharmaceutical development and process analysis.
USE CASES OF AI AND DIGITIZATION IN ANALYTICAL DEVELOPMENT
Leveraging Internal Datasets and Machine Learning to Design Better Biologics
Kadina Johnston, PhD, Senior Specialist, Discovery Biologics, Merck & Co., Inc.
High-quality datasets are key to successful biologics design and pre-developability prediction. Automated collection and curation of pre-developability data from electronic lab notebooks enables rapid retraining and testing of predictors, and it has also been used to direct model-focused data-collection efforts. Furthermore, we use internal data to augment and validate models trained on publicly available datasets, increasing the impact of these models by improving their predictivity on internal assays.
Modular Multi-Objective Optimization Methods for Engineering Functional Proteases with High Therapeutic Potential
Jung-Eun (June) Shin, PhD, Machine Learning Scientist, Seismic Therapeutic
The development of biologic therapeutics is an exacting process, from discovery of a protein with desired function to optimizing for developability. To accelerate this process, we develop a modular multi-objective optimization method that synergistically harnesses deep learning and statistical models combined with structure-based and data-driven rational design. We apply this method to engineer immunoglobulin degrading proteases as potential therapeutics with desired target specificity and potency, low immunogenicity, and favorable manufacturability.
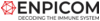
Nicola Bonzanni, Founder & CEO, ENPICOM
We will discuss the key challenges in creating and deploying machine learning for biologics discovery. While creating complex models for discovery and development is becoming commonplace, managing the entire ML model lifecycle is essential for effective use in therapeutic research and maximizing AI investment returns. Discover how a unified platform can streamline AI use in biologics discovery, from model training to consumption.
3:20 pmNetworking Refreshment Break
4:05 pmTransition to Plenary Keynote Session
PLENARY KEYNOTE SESSION
The Role of Protein Engineering in Developing New Innovative Modalities
Puja Sapra, PhD, Senior Vice President, Head R&D Biologics, Engineering and Oncology Targeted Discovery, AstraZeneca
Advances in protein engineering technologies have revolutionized biologics design, paving the way for new innovative drug modalities. This talk will highlight key advancements in the field of protein engineering that have enabled these new modalities to enter the clinic and provide benefit to patients. The talk will also explore the impact of machine learning-enabled deep screening technology on hit identification, lead optimization and development of antibody-based therapies.
YOUNG SCIENTIST KEYNOTE
Antibody-Lectin Chimeras for Glyco-Immune Checkpoint Blockade
Jessica C. Stark, PhD, Underwood-Prescott Career Development Professor, MIT
Despite the curative potential of cancer immunotherapy, most patients do not benefit from treatment. Glyco-immune checkpoints-interactions of cancer glycans with inhibitory glycan-binding receptors called lectins-have emerged as prominent mechanisms of resistance to existing immunotherapies. I will describe development of antibody-lectin chimeras: a biologic framework for glyco-immune checkpoint blockade that is now moving toward the clinic.
5:55 pmWelcome Reception in the Exhibit Hall with Poster Viewing
-jpg_763e004d-1a72-4a71-8e72-41737f8bc448.jpg)
7:20 pmClose of Day
Tuesday, May 13
7:30 amRegistration and Morning Coffee
MENTORING MEET-UP
Creating and Fostering a Productive and Effective Mentor-Mentee Relationship
This meet-up is designed for senior scientists that are interested in becoming a mentor for junior scientists: IN-PERSON ONLY
- What it takes to be a mentor
- Finding the right match
- Goal of Mentoring is to provide support for professional career development and informal coaching
- The Mentor:Mentee relationship: you get out of it what you put into it.
- Establishing boundaries and clear action items to make the most of the experience.
CHALLENGES AND SOLUTIONS
Streamlining Science: The Role of the Digitally Enabled Scientist
Brett Rygelski, Scientist, Pharmaceutical Sciences, Pfizer
Explore the benefits of being a hybrid scientist in my journey from bench scientist to digital practitioner. Discover how digital skills empower the creation of quick, effective solutions, reducing reliance on external experts and manual processes. Learn about the future impact these tools have on portfolio progression through regulatory filings. Understand the importance of fostering digital development opportunities for future scientists.
Empowering Hybrid Scientists: Bridging Lab Expertise and Data Science, a Case Study of Developing an Automated ELN Data Quality Monitoring Tool
This presentation explores the benefits of strategic partnership between the Quantitative Science & Digital Transformation team and the Bioassay Center of Excellence to foster skills of the future at Bristol Myers Squibb. We will share insights on the trainings, rotation experience, key learnings, and projects undertaken. The development of an automated ELN data-quality monitoring tool highlighting the importance of interdisciplinary collaboration in modern research will be presented.
Digital Transformation of Bioprocess Development Labs
Diana Bowley, PhD, Associate Director, CMC Data & Digital Strategy, Bioprocess Development, AbbVie, Inc.
Bioprocess Development groups face challenges with complex modalities, faster development cycles, and more experimental data from HT and PAT technologies. Historically, lab experimental data is dispersed in many different instrument software and unstructured files formats requiring substantial manual data manipulation efforts for experimental insights, decision making, process modeling, and tech transfer. Here we will share our journey to build and deploy a fit-for-science digital ecosystem within our bioprocess development labs.
10:05 amSponsored Presentation (Opportunity Available)
10:35 amCoffee Break in the Exhibit Hall with Poster Viewing
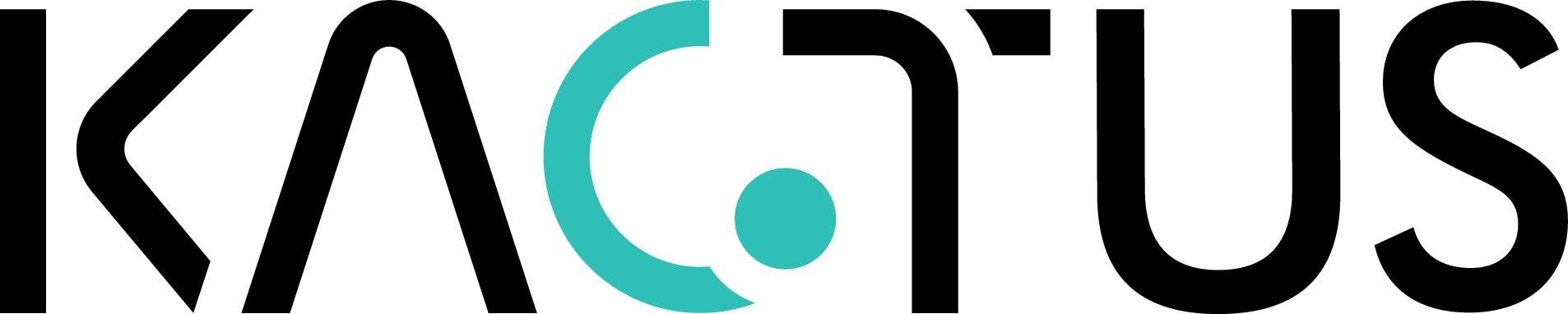
Predicting Viscosity in Concentrated Antibody Solutions Using Machine Learning and Large-Scale Datasets
Pin-Kuang Lai, PhD, Assistant Professor, Chemical Engineering and Materials Science, Stevens Institute of Technology
We measured the viscosity of a large panel of 229 mAbs to develop predictive models for high-concentration screening. We developed DeepViscosity, consisting of 102 ensemble models to classify low-viscosity and high-viscosity mAbs at 150 mg/mL, using a sequence-based DeepSP model. Two independent test sets, comprising 16 and 38 mAbs, were used to assess DeepViscosity’s generalizability. The model exhibited an accuracy of 87.5% and 89.5% on both test sets, respectively.
Elucidation of CHO Cell Metabolism Using Multi-Omics
J. Castro, PhD, Senior Scientist, Cell Engineering & Analytical Sciences, Johnson & Johnson Innovative Medicine
This study investigated the metabolism of Chinese Hamster Ovary (CHO) cells through a multi-omics approach, integrating proteomic and metabolomic datasets. By developing genome-scale models, metabolic patterns were successfully identified and analyzed, leading to new hypotheses about the regulatory mechanisms driving cellular behavior. These findings provided insights that enhance the understanding of CHO cell metabolism and may improve strategies for optimizing protein bioproduction.
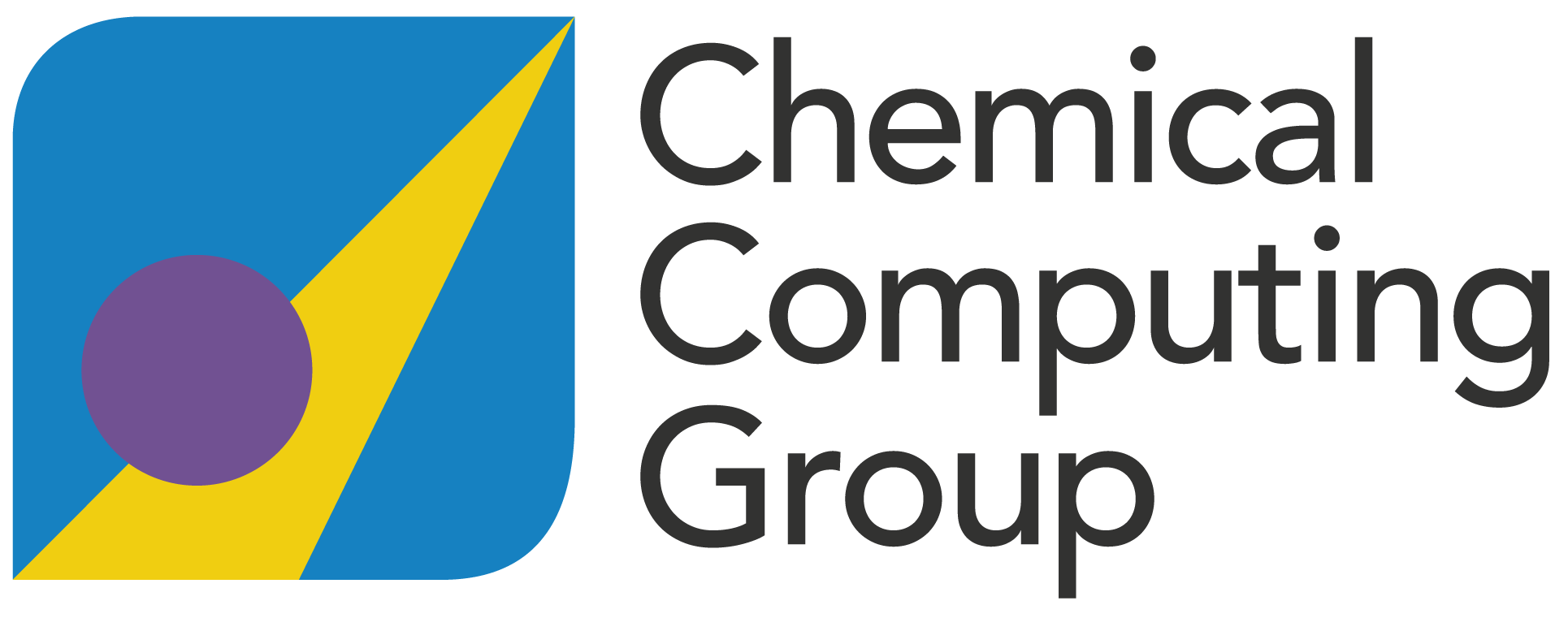
Nels Thorsteinson, Director of Biologics, Biologics, Chemical Computing Group
We present a method for modeling antibodies and performing pH-dependent conformational sampling, which can enhance property calculations. Structure-based charge descriptors are evaluated for their predictive performance on recently published antibody pI, viscosity, and clearance data. From this, we devised four rules for therapeutic antibody profiling which address developability issues arising from hydrophobicity and charged-based solution behavior, PK, and the ability to enrich for those that are approved by the FDA.
12:30 pmSponsored Presentation (Opportunity Available)
12:45 pmSession Break
12:50 pmLuncheon Presentation (Sponsorship Opportunity Available) or Enjoy Lunch on Your Own
1:50 pmClose of ML and Digital Integration in Biotherapeutic Analytics Conference
6:30 pmRecommended Dinner Short Course
SC6: Developability of Bispecific Antibodies
*Separate registration required. See short course page for details.
* 不測の事態により、事前の予告なしにプログラムが変更される場合があります。
アジェンダ・講演者・スポンサー更新
2025年 プログラム
表示する:
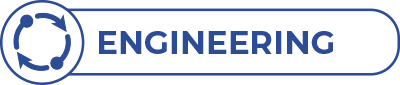
工学ストリーム
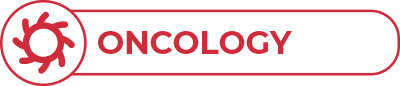
腫瘍ストリーム
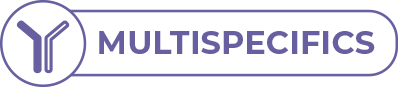
多重特異性ストリーム
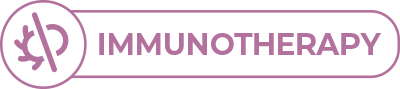
免疫療法ストリーム
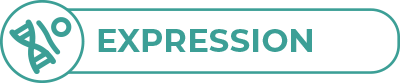
発現ストリーム
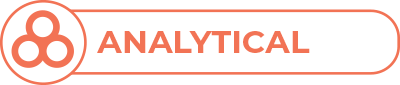
分析法ストリーム
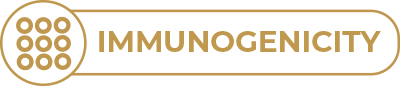
免疫原性ストリーム
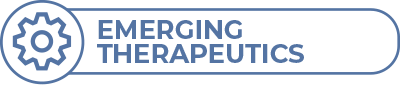
新興治療ストリーム
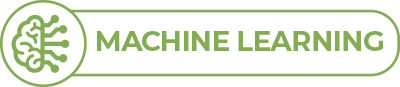
機械学習ストリーム