Cambridge Healthtech Instituteの第4回年次
Machine Learning Approaches for Protein Engineering
タンパク質工学向け機械学習アプローチ
Putting Theory into Practice and Streamlining Biologic Development
理論の実践とバイオロジクス開発の合理化
2025年5月15日 - 16日 EDT(米国東部標準時・夏時間)
Scientific Advisory Board
M. Frank Erasmus, PhD, Head, Bioinformatics, Specifica, an IQVIA business
Victor Greiff, PhD, Associate Professor, University of Oslo and Director of Computational Immunology, IMPRINT
Maria Wendt, PhD, Global Head and Vice President, Digital and Biologics Strategy and Innovation, Sanofi
Sunday, May 11
1:00 pmMain Conference Registration
2:00 pmRecommended Pre-Conference Short Course
SC1: In silico and Machine Learning Tools for Antibody Design and Developability Predictions
*Separate registration required. See short course page for details.
Thursday, May 15
7:45 amRegistration and Morning Coffee
FURTHER ADVANCES ON ML/DL: NOVEL PLMs, CODON-BASED PLMs, INTERPRETABILITY, DIFFUSION
KEYNOTE PRESENTATION: Generative AI to Accelerate Prediction and Design in Biomedicine and Sustainability
Debora S. Marks, PhD, Associate Professor, Systems Biology, Harvard Medical School
There’s now an amazing opportunity to accelerate discovery across important 21st century challenges by using computation tightly coupled to biological experiments and clinical medicine. I will describe some recent approaches from my lab for these challenges where we have developed new machine learning methods that can exploit the enormous natural sequence diversity and our ability to sequence DNA at scale. To demonstrate the power of these new approaches I will present recent work predicting the effects of human genetic variation on disease and drug response, anticipation of viral escape from the host immune system for vaccine design, protein design for enzyme optimization, antibodies and sustainable biomaterials.
Large Language Models for mRNA Design
Sven Jager, PhD, Lead, Computational Science, Sanofi Germany GmbH
mRNA-based vaccines and therapeutics are increasingly popular and used for a variety of conditions. A key challenge in designing these mRNAs is sequence optimization. Even small proteins or peptides can be encoded by a vast number of mRNA sequences, each affecting properties such as expression, stability, and immunogenicity. To facilitate the selection of optimal sequences, we developed CodonBERT, a large language model (LLM) specifically for mRNAs.
Progress Report on AlphaFold and OpenFold-Driven Biomolecular Modeling
Nazim Bouatta, PhD, Senior Research Fellow, Lab of Systems Pharmacology and Systems Biology, Harvard Medical School
AlphaFold2 has transformed structural biology with groundbreaking advances in protein structure prediction. However, despite these advances, many challenges remain. In this talk, I will present a progress update on AlphaFold2 and share insights gained from OpenFold, an optimized and trainable variant of AlphaFold2. I’ll also explore potential paths to address the limitations of AlphaFold-like systems.
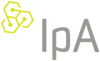
Shuji Sato, Senior Director Client Relations, ImmunoPrecise Antibodies
This session will explore effective strategies for accelerating lead selection from a diverse panel of antibodies. Key techniques presented include proprietary methods for leveraging the unique immune system of rabbits, early epitope landscape profiling, and the use of IPA's in silico-driven diversification and optimization workflows, resulting in the rapid delivery of optimized antibodies ready for clinical development.
10:15 amSponsored Presentation (Opportunity Available)
10:30 amCoffee Break in the Exhibit Hall with Poster Viewing
11:15 amTransition to Plenary Fireside Chat
PLENARY FIRESIDE CHAT
Riding the Next Biotech Wave-Trends in Biotech Investments, Partnering, and M&As
Jakob Dupont, MD, Executive Partner, Sofinnova Investments
- Emerging Biotherapeutic Modalities, Technologies and Innovations- ADCs, radiopharmaceuticals, GLP-1, AI, machine learning, and other exciting trends to watch
- Introduction to different strategies for investments, M&As, partnering, licensing etc.
- Investing in platforms versus assets
- Advice on funding options for start-ups, early to late stage clinical programs, etc.
12:25 pmLuncheon in the Exhibit Hall and Last Chance for Poster Viewing
DEVELOPABILITY AND OPTIMIZATION
Biophysical Cartography of the Native and Human-Engineered Antibody Landscapes Quantifies the Plasticisity of Antibody Developability
Victor Greiff, PhD, Associate Professor, University of Oslo and Director of Computational Immunology, IMPRINT
Developing effective monoclonal antibody (mAb) therapies requires optimizing multiple properties, known as 'developability,' to ensure they can progress through the development pipeline. However, there is limited understanding of how the characteristics (redundancy, predictability, sensitivity) of developability parameters (DPs) in human-engineered antibodies compare to those in natural antibodies. We analyzed 86 DPs across two million antibody sequences, finding key differences in the predictability and sensitivity of sequence- and structure-based DPs. Our findings reveal that human-engineered antibodies occupy a narrower space within the natural antibody landscape, offering a foundation for more precise mAb design.
Machine Learning-Guided Selection and Design Optimization of Chemically Stable Antibodies
Saeed Izadi, PhD, Senior Principal Scientist & Group Leader, Pharmaceutical Development, Genentech, Inc.
Chemical degradation poses significant risks in the developability of antibodies, potentially leading to loss of binding or liabilities like aggregation and immunogenicity. Here, I will present a machine-learning-driven approach to identify structural features crucial for chemical stability. I will share experimental evidence showing that targeted point mutations can effectively mitigate chemical liabilities without compromising binding, and discuss how these insights can inform the multi-parameter optimization of antibodies for enhanced stability.
Insights from the AIntibody Benchmarking Competition
M. Frank Erasmus, PhD, Head, Bioinformatics, Specifica, an IQVIA business
This talk highlights the integration of strategic data collection and intelligent experimental design to advance AI-powered antibody discovery and optimization. We will share updates from the AIntibody competition, a benchmarking initiative engaging the biotech, pharma, academia, and AI communities. These efforts, alongside tailored discovery campaigns for individual collaborators, aim to accelerate innovation and drive progress in early-stage therapeutic discovery.
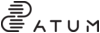
As the demand for high-quality biologics accelerates, biopharmaceutical developers face increasing pressure to optimize cell line development for speed, quality, stability, and scalability. ATUM’s Leap-In Transposase® technology is transforming the landscape of protein expression by enabling rapid, predictable, and high-yield stable cell line generation. Unlike traditional random integration methods, the Leap-In Transposase® system facilitates highly efficient transgene integration at transcriptionally active loci, leading to enhanced expression, improved genetic stability, and accelerated development timelines. In this presentation, we will showcase how Leap-In Transposase® technology is empowering biopharma teams to achieve faster workflows while eliminating the need for labor-intensive single-cell cloning. We will present case studies demonstrating its impact across monoclonal antibodies, multispecifics, and novel therapeutic proteins, highlighting significant improvements in titers, scalability, and manufacturability. Furthermore, we will discuss its seamless integration with CHO and HEK cell systems, making it an ideal solution for both early-stage discovery and commercial-scale production.
4:00 pmNetworking Refreshment Break
Generative AI-Guided Design of Vaccine Immunogens
Reda Rawi, PhD, Staff Scientist and Co-Head, Structural Bioinformatics Core, NIH NIAID
Structure-based vaccine design campaigns that aim to stabilize full-length proteins will not succeed when the virus is evading immune response by sequence diversity. In this work, we capitalized on the recent major advanced that have been achieved in protein design using generative AI tools. We in silico designed de novo proteins that scaffold sequence-conserved epitope regions of the antigens of interest. Next, we incorporated the in silico designed mini proteins onto self-assembling nanoparticles and performed pre-clinical animal immunization studies eliciting immune responses.
AbGPT: De novo Antibody Design via Generative Language Modeling
Amir Barati Farimani, PhD, Associate Professor, Machine Learning, Carnegie Mellon University
The adaptive immune response relies on B-cell receptors (BCRs) for pathogen neutralization, yet designing BCRs de novo remains challenging due to structural complexity. Here, we introduce Antibody Generative Pretrained Transformer (AbGPT), a fine-tuned model from a foundational protein language model. Using a tailored generation and filtering pipeline, AbGPT generated 15,000 high-quality BCR sequences, effectively capturing the intrinsic variability and conserved regions critical to antibody design.
Scaling Foundation Models for Protein Generation
6:00 pmClose of Day
Friday, May 16
7:15 amRegistration Open
INTERACTIVE DISCUSSIONS
Interactive Discussions are informal, moderated discussions, allowing participants to exchange ideas and experiences and develop future collaborations around a focused topic. Each discussion will be led by a facilitator who keeps the discussion on track and the group engaged. To get the most out of this format, please come prepared to share examples from your work, be a part of a collective, problem-solving session, and participate in active idea sharing. Please visit the Interactive Discussions page on the conference website for a complete listing of topics and descriptions.
Delivering on the AI Antibody Promise: The AIntibody Benchmarking Competition
- AI promises in antibody discovery and optimization: will they really revolutionize the field? or just another way of addressing solved problems?
- What can AI do now? And where are we seeing the greatest value relative to existing technologies?
- The AIntibody benchmarking competition: Did AI deliver on the AIntibody challenges?
- Ideas for future benchmarking competitions
BENCHMARKING AND AUTOMATION
Closed-Form Test Functions for Biophysical Sequence Optimization Algorithms
Samuel Stanton, PhD, Machine Learning Scientist, Prescient Design, Computational Sciences, Genentech
Many researchers are trying to replicate the success of machine learning (ML) in computer vision and natural language processing in modeling biophysical systems. As a discipline, ML heavily relies on low-cost empirical benchmarks to guide algorithm development, but available benchmarks for biophysical applications have major shortcomings. Drawing inspiration from mutational landscape models, we propose Ehrlich functions, a new class of test functions for biophysical sequence optimization algorithms.
Avoiding Pitfalls in ML Model Validation for Protein Design: The Importance of Data Splits
Norbert Furtmann, PhD, Head, Computational and High-Throughput Protein Engineering, Large Molecule Research, Sanofi
This presentation will explore the development of machine learning models for protein property prediction. Focusing on customized protein language models for the NANOBODY modality, it will demonstrate the implementation of a downstream thermostability predictor. Using this example, the talk will emphasize the critical importance of meaningful data splits in model training and validation.
Closing the Loop: Ultra-Fast Wet Lab Validation for AI-Guided Protein Design
Julian Englert, MS, Co-Founder and CEO, Adaptyv Biosystems
Adaptyv accelerates data generation for training and validating AI models with a high-throughput lab that companies can access via our software interface and API. We empower protein-design teams to validate their AI models many times faster than before, without the need to run in-house wet labs. We're partnering with dozens of companies-from techbio startups to major pharma-and generated lab data for thousands of novel proteins.
10:30 amNetworking Coffee Break
End-to-End Antibody Discovery against 100s of Targets per Year: From Antigen to AI-Driven Insights
André A. R. Teixeira, PhD, Senior Director, Antibody Platform, Institution for Protein Innovation
At IPI, we perform 300 antibody and VHH discovery campaigns per year against human targets. Our end-to-end antibody discovery platform integrates antigen production, yeast display libraries (Fab and VHH), next-generation sequencing, antibody production, and biophysical characterization. With a success rate exceeding 85%, this platform generates antibodies that benefit the community. Also, the data sets offer powerful opportunities for AI and machine learning applications, driving innovation in antibody discovery and development.
The IMMREP TCR-Epitope Prediction Challenge: Lessons Learned and Future Directions
Justin Barton, PhD, AI Scientist, Xaira Therapeutics
The IMMREP23 competition evaluated TCR-pMHC interaction prediction methods from 53 participating teams submitting 398 sets of predictions. Results showed reasonable performance for "seen" pMHC targets but near-random performance for "unseen" peptides, highlighting an unsolved generalization challenge. Here we discuss what has been learned from detailed analysis of predictions and provide insights for improving future benchmarks by carefully addressing biases in dataset construction.
DALSA-DTU Arena for Life Science Automation
Timothy Patrick Jenkins, PhD, Assistant Professor and Head, Data Science, DTU Bioengineering
The DTU Arena for Life Science Automation (DALSA) is a pioneering initiative aimed at advancing the technologies and use of automation within Life Science R&D and manufacturing for academia as well as industry. Our mission is to establish a central and open access hub for technology development and educational advancement in life science automation, serving as a test bed for the full-scale implementation of DALSA. With successful multi-disciplinary collaborations between academia and industry and involving electrical, mechanical, bio, and computational engineers, we are already seeing the benefits of a centralised open access automation hub.
12:30 pmClose of Summit
* 不測の事態により、事前の予告なしにプログラムが変更される場合があります。
アジェンダ・講演者・スポンサー更新
2025年 プログラム
表示する:
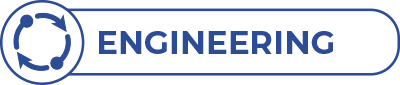
工学ストリーム
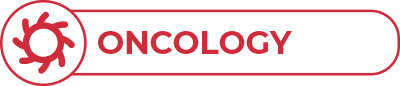
腫瘍ストリーム
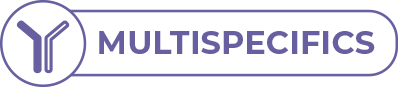
多重特異性ストリーム
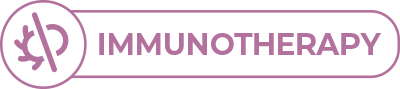
免疫療法ストリーム
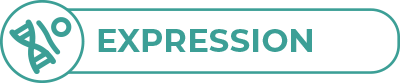
発現ストリーム
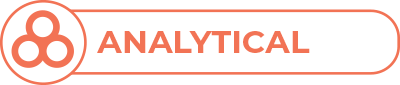
分析法ストリーム
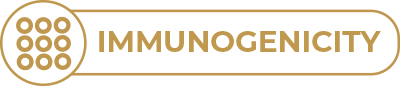
免疫原性ストリーム
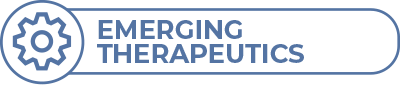
新興治療ストリーム
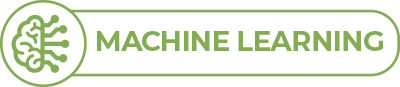
機械学習ストリーム